Research
Engineering medicine to save lives and ensure good health of future generations.
Research
Engineering medicine to save lives and ensure good health of future generations.
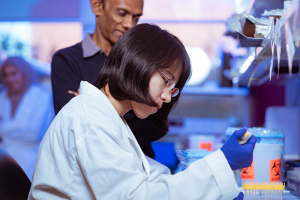
DISCOVER • INNOVATE • ACCELERATE
Combining engineering and medicine to advance scientific discoveries, medical interventions, health, diagnosis, treatment, therapies, and medical technologies; we are engineering life sciences in ways never done before.
Research Excellence
At the interface of biology and nanoscience/nanotechnology, faculty research in this domain encompasses new biomaterials, micro- and nanotechnology-…
Learn more
Medical imaging, the science and engineering of creating visual representations of the interior and parts of the human body for clinical analysis and…
Learn more
Neural Engineering, also known as Neuroengineering, encompass the techniques to understand, repair, replace, or enhance neural systems. Our faculty…
Learn more
INDUSTRIAL RELATIONS
Learn more about the various ways you can partner with us.
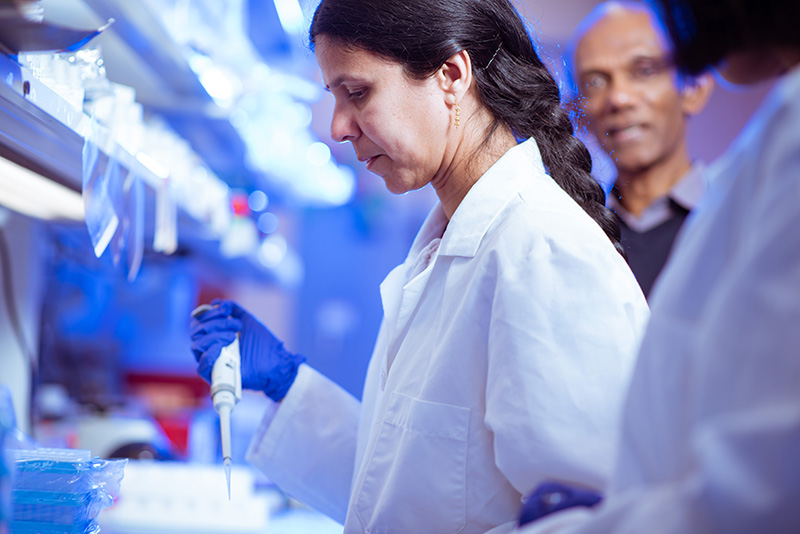
Theses and Dissertations
Learn more about our masters and PhD students and their research topics.